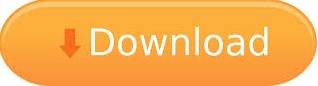
It is also uncommon for libraries to support visualizing a specific feature vector as it weaves down through a tree's decision nodes we could only find one image showing this.
For example, we couldn't find a library that visualizes how decision nodes split up the feature space. Unfortunately, current visualization packages are rudimentary and not immediately helpful to the novice. Visualizing decision trees is a tremendous aid when learning how these models work and when interpreting models. Discussionĭecision trees are the fundamental building block of gradient boosting machines and Random Forests(tm), probably the two most popular machine learning models for structured data.
Take a look in notebooks! Here we have a specific notebook for all supported ML libraries and more. Jump right into the examples using this Colab notebook We welcome info from users on how they use dtreeviz, what features they'd like, etc.
Visualize decision tree python how to#
See How to visualize decision trees for deeper discussion of our decision tree visualization library and the visual design decisions we made. of San Francisco, where he was founding director of the University of San Francisco's MS in data science program in 2012.
Terence Parr, a tech lead at Google and until 2022 was a professor of data science / computer science at Univ. With 1.3, we now provide one- and two-dimensional feature space illustrations for classifiers (any model that can answer predict_probab()) see below. Currently supports scikit-learn, XGBoost, Spark MLlib, and LightGBM trees. Below is the example of the markdown report for Decision Tree generated by mljar-supervised.Dtreeviz : Decision Tree Visualization DescriptionĪ python library for decision tree visualization and model interpretation. I add this limit to not have too large trees, which in my opinion loose the ability of clear understanding what’s going on in the model. One important thing is, that in my AutoML package I’m not using decision trees with max_depth greater than 4. You can check the details of the implementation in the github repository. I’m using dtreeviz package in my Automated Machine Learning (autoML) Python package mljar-supervised. It would be great to have dtreeviz visualization in the interactive mode, so the user can dynamically change the depth of the tree. it shows the distribution of the class in the leaf in case of classification tasks, and mean of the leaf’s reponse in the case of regression tasks. it shows the class-color matching legend. it shows the distribution of decision feature in the each node (nice!). feature_names ) vizįrom above methods my favourite is visualizing with dtreeviz package. I will use default hyper-parameters for the classifier.įrom ees import dtreeviz # remember to load the package viz = dtreeviz ( regr, X, y, target_name = "target", feature_names = boston. I will train a DecisionTreeClassifier on iris dataset. Train Decision Tree on Classification Task I will show how to visualize trees on classification and regression tasks. plot with dtreeviz package (dtreeviz and graphviz needed). plot with _graphviz method (graphviz needed).
plot with _tree method (matplotlib needed). print text representation of the tree with _text method. They can support decisions thanks to the visual representation of each decision.īelow I show 4 ways to visualize Decision Tree in Python: In scikit-learn it is DecisionTreeRegressor.ĭecision trees are a popular tool in decision analysis. Regression trees used to assign samples into numerical values within the range. In scikit-learn it is DecisionTreeClassifier. Classification trees used to classify samples, assign to a limited set of values - classes.
The decision trees can be divided, with respect to the target values, into: Decision Tree learning is a process of finding the optimal rules in each internal tree node according to the selected metric. A decision is made based on the selected sample’s feature. In each node a decision is made, to which descendant node it should go. To reach to the leaf, the sample is propagated through nodes, starting at the root node. The target values are presented in the tree leaves. It is using a binary tree graph (each node has two children) to assign for each data sample a target value. A Decision Tree is a supervised algorithm used in machine learning.